Researchers at the University of Ottawa believe the potential of vehicular crowd-sensing extends far beyond navigating roads and highways.
Connected vehicles, they argue, can help the city prioritize pothole repairs, notify first responders to collisions and identify the quickest routes during rush hour.
Burak Kantarci, an associate professor at the University of Ottawa Faculty of Engineering, and his team are focused on Internet of Things and big data analytics, and see an opportunity to take advantage of the visual and digital sensors on connected vehicles to make roads safer.
“Connected vehicles have so much potential,” says Kantarci. “It is a communication hub, sensing server and a data storage unit. It can be a great resource to make our infrastructure work better.”
Potholes slow down traffic and damage vehicles. Researchers have developed intelligent algorithms to collect and analyze crowd-sensed data from various built-in sensors. Crowd-sensed data can also be used to detect potholes, identify which ones are the most urgent and contact the City. Currently, the city relies on residents to report potholes but lacks the ability to objectively determine which potholes are the most dangerous or most disruptive to traffic flow. Kantarci says the city can be more efficient in its approach to pothole repairs by analyzing crowd-sensed data.
Similarly, sensors embedded within connected and autonomous cars can also detect when surrounding vehicles have been involved in a collision. Kantarci and his team are currently analyzing various accident images to assess the severity of a crash by using deep neural networks in order to figure out which first responders are needed, and in what number. They want to extend their current work to develop integrated solutions to analyze photos taken by a vehicle
“When there’s a car accident, police, ambulance and fire trucks show up,” says Kantarci. “Sometimes you only need police. If we can figure out who should respond, that will free up resources and cause less traffic.”
5G networks
To collect and preprocess visual data, we will need lots of communication and computing power. Kantarci says new 5G networks will make acquiring the data quicker, and this will consequently enable accurate
on-the-fly decisions.
Another advantage to crowd-sensed data is real-time analytics of traffic patterns, road conditions and weather. This data can be used to determine the safest and quickest exit routes to get out of rush-hour traffic, as well as direct motorists during emergencies such as major flooding. Kantarci’s team developed an algorithm for ambulances that can optimally switch between alternate routes considering the traffic and the situation of a patient.
The major challenge to utilizing this data is making sure there’s enough processing power. The terabytes of data collected from millions of connected devices will need to be stored in the cloud. Not all the data will need to be analyzed in real time, but can be stored for future uses.
There’s also the environmental and operational impact. Large amounts of data are stored, processed and distributed through data centres, which use large amounts of energy. Kantarci and his team have also designed energy efficient communication protocols for IoT-fog systems.
“We’re still going to need data centres but we want something that’s flexible, close to the end user and cost effective,” he says.
Learn more about uOttawa’s research at engineering.uOttawa.ca.
Visualizing big data
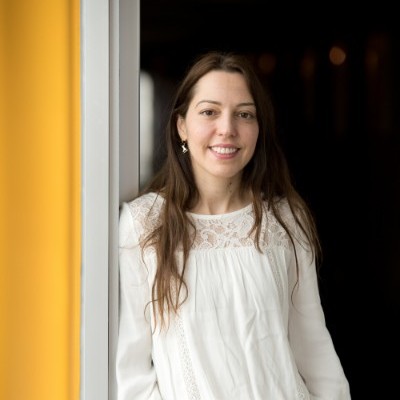
Researchers at the University of Ottawa Faculty of Engineering that can interpret inputs from virtually any information source.
Typically, visualization systems such as GPS are designed based on the type of data. Associate professor Verena Kantere and her team created a system, which implements novel algorithms and techniques, which can visualize any type of large linked dataset in an interactive chart.
Kantere says most visualization systems must pre-load data onto a device in order to work. For example, a user would find it difficult to zoom in on individual streets in a map showing a province’s interconnected transportation system. That information wouldn’t be pre-loaded so the processing time would be slow. For large datasets, such systems require a prohibiting amount of memory and rely on expensive infrastructure failing to scale for multiple users or machines with limited computational resources.
Other systems, in order to cope with large datasets use sampling and aggregation techniques to visualize what they interpret as important information or visualize a limited number of elements based on the user requests. While such systems have few requirements
regarding the dataset, they present a limited part of the information to the user hindering the overall understanding of the available information.
“Many diverse types of datasets consist of interrelated data elements like links on a Wikipedia page,” says Kantere. “It’s very hard to make that efficiently interactive without preprocessing the data.”
The solution is to pre-process the data beforehand, and link related data elements together.
There are also tradeoffs. A visual program tracking Ottawa traffic could be accurate, showing traffic patterns for every street but might not be so fast.
“State-of-the-art systems can’t offer both,” says Kantere. “We want to see how far we can go.”