The emergence and availability of Generative AI Applications (most notably ChatGPT and its incorporation into BING AI) has heightened curiosity about the potential of Artificial Intelligence (AI). Applications of AI in business contexts are countless and can supercharge digital strategies and add tremendous value (and have been doing so for years).
At the same time, there is risk of confusion as media frenzy, and sometimes pseudo-scientific hype, obscures practical applications of the technology, current limitations, and likely risks.
In a recent HBR article “The AI Hype Cycle Is Distracting Companies,” Eric Siegel, an expert in Machine Learning, argues the hype and terminology, namely the word “intelligence,” can lead to disillusionment and lost opportunities.
To overcome some of this confusion, here are some practical considerations for delivering business value from AI applications.
It’s important to note, I am using the term AI to refer to diverse technologies including Natural Language Processing, Machine Vision, Deep Learning and Machine Learning. It is also worth noting that ISO/IEC 22989 defines an AI System as an “engineered system that generates outputs such as content, forecasts, recommendations, or decisions for a given set of human-defined objectives.”
Strategy and business applications of AI
Business applications for AI technology are as vast as opportunities for automation, decision support, and customer engagement and can touch on every functional area of an organization including supply chain management, human resources, engineering, IT help desk, customer support, and quality assurance.
Given the range of opportunities, directing AI investments through a business strategic lens is important. If not integrated into strategy, AI investments can become fragmented and unfocused, or organizations can fail to act.
AI adoption
Narrow AI (built for a specific purpose) has been utilized by large organizations and part of our lives for many years, ranging from Netflix personalized suggestions to Google Photos. Yet there is still significant unexploited opportunity in business applications especially in medium–sized organizations.
A recent KPMG survey noted that only 35 per cent of Canadian organizations currently use AI in their operations compared with 72% in the US. Companies that have adopted AI in some way in their operations also recognize that they have only scratched the surface of the potential of these opportunities.
Supercharging strategy with AI
I think of opportunities in AI as loosely falling into two buckets.
One bucket is productivity solutions that help an organization perform general business functions in more automated ways. Business leaders can benefit from scans of the multitude of tools that are increasingly incorporating AI and automation.
Examples of those applications include augmenting the IT help desk, prescreening candidates, testing, automated visual inspections, and automating document classification.
The second bucket of opportunities are the applications of AI that solve core business problems to advance key strategies for the organization. This bucket likely contains higher return on investment opportunities where business transformation and business differentiation can supercharge strategy.
These opportunities demand an understanding of “the art of the possible” in AI, coupled with visionary business strategy. There are many AI technology organizations competing to provide industry specific solutions which business leaders should seek to become familiar with.
A first-hand strategic example
One such example is an organization that is trying to solve a customer retention question central to its strategy.
Several years ago, I supported an organization with the identification and implementation of an AI tool which utilized natural language processing to read hundreds of thousands of responses from customer feedback surveys and interactions.
The organization needed to gain deeper insights into customer retention. We implemented a SaaS (Software as a Service) solution which came with an enterprise–ready foundational model for customer engagement designed to detect negative and positive sentiment and classify feedback topically.
This solution made it possible to implement and gain insights in around three months for a medium-sized non-technology organization that could not curate large data sets or build its own algorithms.
The model could then be tuned to the specific context as organization–specific data was fed into the model. Further to this, the insights derived from this tool were integrated into the pre-existing continuous improvement mechanisms to enable thousands of employees with these insights to improve customer satisfaction.
Data, data, data and readiness
Data is critical when it comes to AI. Machine learning models need to be trained using quality data. Usama Fayyad, CEO of Open Insights, and a pioneer in this field notes: “For data to be adequately utilized it must be clean, detailed, granular, and accurately labeled for training AI algorithms.”
The importance of having the right data cannot be understated. In my experience, clients will often realize they need a data strategy to support digital AI strategies. Many AI projects will incorporate work around data.
Beyond data, leaders will need to consider other areas of readiness to enable successful adoption of AI technologies. Another key is talent, people, and upskilling. People who understand and utilize AI are going to be in a better position than those who do not and so will the organizations that employ them.
Risk management and AI
Identifying the most pertinent risks of AI to an organization will allow for their mitigation through various means including education and governance.
Common areas of risk to consider include false information based on imperfect data training sets, greater cybersecurity risks due to use of AI in phishing and hacking, and data ownership and intellectual property considerations.
It is also important to consider that the risk of inaction while these technologies accelerate can leave organizations behind.
Why AI?
Finally, there is a business fundamental that does not change, which is to start with the “why”. To be successful, leaders need clarity around the opportunity to be captured or the problem that needs to be solved and its relevance to AI.
Where are costly and painful frictions in business operations? What external pressures are created by AI on core products or services? What differentiating advantage can AI offer?
This takes us back to the beginning of this blog and the importance of directing AI investments through a business strategic lens. Most importantly, action orientation is required given the pace of change.
AI opportunities & considerations
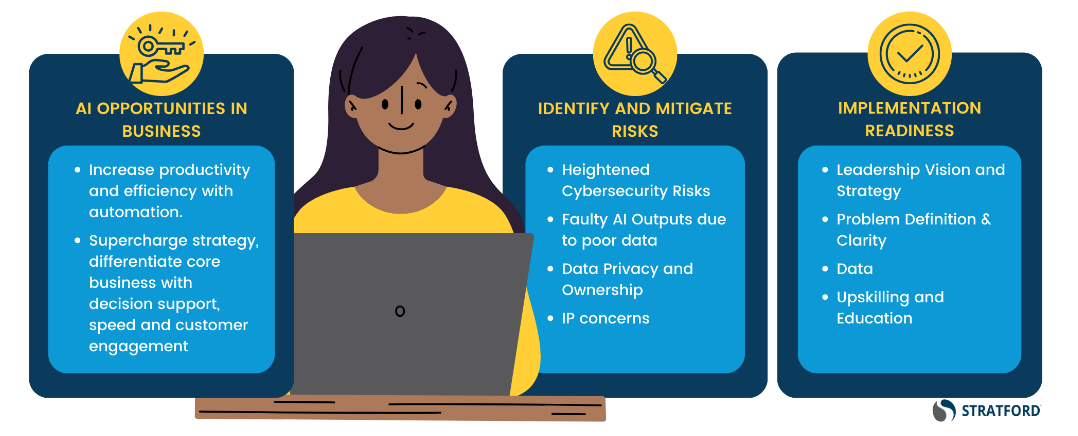
About Rana Chreyh:
A senior executive, professional engineer, and Ivy league executive MBA business graduate with over 25 years of innovation in technology and business domains.
Rana’s experience includes strategy development and implementation and large-scale solution delivery including for not-profit, technology start-ups and global fortune 500 companies.
Rana Chreyh is recognized for her ability to seamlessly transverse and integrate business and IT strategy due to her broad and deep experience with industry expertise in technology, medical devices, HealthTech and healthcare.
As Practice Leader, Management Consulting at Stratford, Rana will bring the team’s expertise to the table to meet your specific needs.